HOLO 3: The Research Partner
“My Research Partner would have to pose a real challenge to my own thinking. They’d sit outside of the inertia that can set in, as a field of inquiry and a mode of practice becomes known well, lauded, praised.”
Peli Grietzer, strolling through Berlin Tiergarten during peak lockdown. “I was going through a rough week and my artist friend Stanton Taylor, who took the picture, invited me on a walk,” Grietzer tells HOLO. “We found this cool tree that we would like to believe was struck by lightning.”
“This is when I thought of Peli Grietzer, a brilliant scholar, writer, theorist, and philosopher, whose work borrows from machine learning theory to think through ‘ambient’ phenomena like moods, vibes, and styles.”
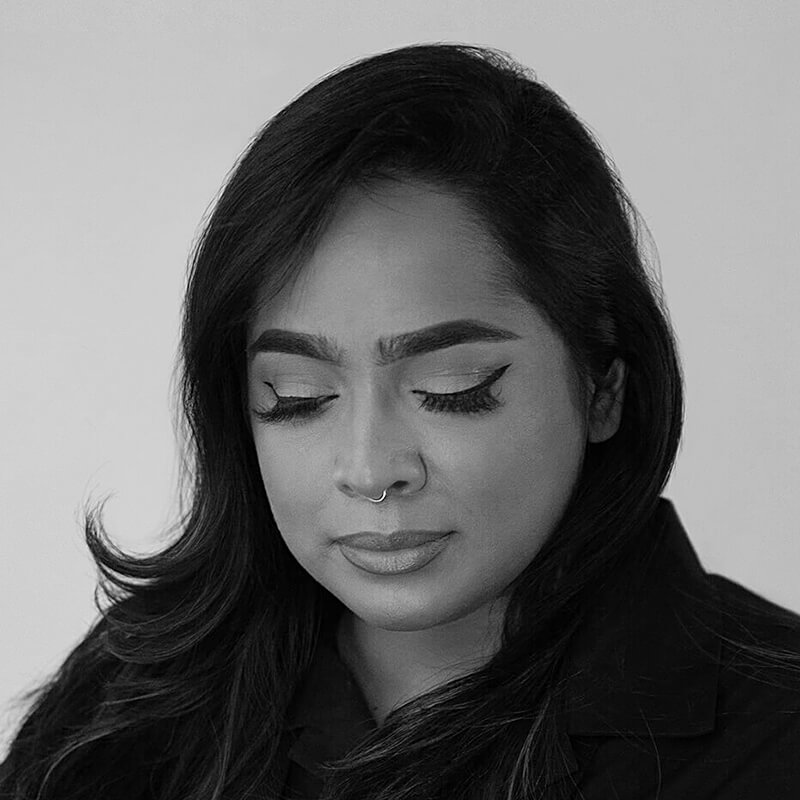
Nora N. Khan is a New York-based writer and critic with bylines in Artforum, Flash Art, and Mousse. She steers the 2021 HOLO Annual as Editorial Lead
Each issue asks for the guest editor to choose a Research Partner, an interlocutor “who brings niche expertise and a unique perspective to the table.” I took this to mean a person who will be able to engage with the emerging frame, then provide feedback in a few sessions, as the magazine takes form. I first listed all my dream conversationalists, whose research and thinking I was drawn to. This list was long. It covered many possible expressions of research, from experimental and solitary to collaborative, hard scientific to qualitative and artistic research, computational to traditional archival research. There were literary researchers, Twitter theory-pundits, long-standing scholars in all the decades of experiments-in-art-and-technology, curators and editors who ground their arguments in original research.
As my editorial frame evolved into four distinct prompts, it seemed clear that the Partner would also need to confidently, easily critique the frame. They’d ideally provide prompts and encouragement from their perspective, expertise, practice, and scholarship,to help push the frame and broaden it.
I also realized that the HOLO Research Partner would have to pose a real challenge to my own thinking, and counter the clear positions and takes that come from being too far in (too far gone?) a dominant critical discourse about technological systems, which can often speak to itself (See: opening note). They’d sit outside of the inertia that can set in, as a field of inquiry and a mode of practice becomes known well, lauded, praised. (I think, here, of calls in which funders ask for guidance to “the most innovative work being done of all the innovative work being done in art and technology.”) As I wrote in my opening letter, this year has so swiftly turned us to embrace what we want to hear, take in, to what we still hunger to explore.
This is really when I thought of Peli Grietzer, a brilliant scholar, writer, theorist, and philosopher based in Berlin. Peli received a PhD from Harvard in Comparative Literature under the advisorship of Hebrew University mathematician Tomer Schlank. Peli’s work borrows mathematical ideas from machine learning theory to think through the ontology of “ambient” phenomena like moods, vibes, styles, cultural logics, and structures of feeling. Peli also contributes to the experimental literature collective Gauss PDF, and is working on a book project expanding on their “sometimes-technical” research, as they call it, entitled Big Mood: A Transcendental-Computational Essay on Art. He is also working on the artist Tzion Abraham Hazan’s first feature film.
I first ran across Peli’s writing and thinking through his epic erudition in “A Theory of Vibe”, published in 2017 in the research and theory journal Glass Bead. The chapters published were excerpted from their dissertation (which it sounds like they are currently turning into a book). I then virtually met Peli in 2017, over a shaky group call, in which a few Glass Bead editors and contributors to Site 1: The Artifactual Mind, called in from Paris and Berlin. Sitting in New York at Eyebeam on those old red metal chairs, I took frantic notes as Peli spoke. I struggled to keep up. It was exciting to be exposed to such a livewire mind. As it goes in these encounters, I felt my own thinking evolving, sensing, with relief, all the ways literary theory and criticism, and philosophical writing on AI could overlap in a way that many camps could start to speak to one another.
“Sitting in New York at Eyebeam on those old red metal chairs, I took frantic notes as Peli spoke. I struggled to keep up. It was exciting to be exposed to such a livewire mind.”
Excerpt from William Carlos Williams, Paterson, 1927, published alongside Peli Grietzer’s “A Theory of Vibe” in Glass Bead
“I felt my own thinking evolving, sensing, with relief, all the ways literary theory and criticism, and philosophical writing on AI could overlap in a way that many camps could start to speak to one another.”
Most folks have noticed the distinctly generous qualities of Peli’s work, and have an experience reading “A Theory of Vibe,” too. The experience might begin with its opening salvos:
• An autoencoder is a neural network process tasked with learning from scratch, through a kind of trial and error, how to make facsimiles of worldly things. Let us call a hypothetical, exemplary autoencoder ‘Hal.’ We call the set of all the inputs we give Hal for reconstruction—let us say many, many image files of human faces, or many, many audio files of jungle sounds, or many, many scans of city maps—Hal’s ‘training set.’ Whenever Hal receives an input media file x, Hal’s feature function outputs a short list of short numbers, and Hal’s decoder function tries to recreate media file x based on the feature function’s ‘summary’ of x. Of course, since the variety of possible media files is much wider than the variety of possible short lists of short numbers, something must necessarily get lost in the translation from media file to feature values and back: many possible media files translate into the same short list of short numbers, and yet each short list of short numbers can only translate back into one media file. Trying to minimize the damage, though, induces Hal to learn—through trial and error—an effective schema or ‘mental vocabulary’ for its training set, exploiting rich holistic patterns in the data in its summary-and-reconstruction process. Hal’s ‘summaries’ become, in effect, cognitive mapping of its training set, a kind of gestalt fluency that ambiently models it like a niche or a lifeworld.
Through this playful use of Hal, readers are asked to consider and hold the “summaries” Hal makes, the lifeworld it models, to understand how an algorithm learns:
• What an autoencoder algorithm learns, instead of making perfect reconstructions, is a system of features that can generate approximate reconstruction of the objects of the training set. In fact, the difference between an object in the training set and its reconstruction—mathematically, the trained autoencoder’s reconstruction error on the object—demonstrates what we might think of, rather literally, as the excess of material reality over the gestalt-systemic logic of autoencoding. We will call the set of all possible inputs for which a given trained autoencoder S has zero reconstruction error, in this spirit, S’s ‘canon.’ The canon, then, is the set of all the objects that a given trained autoencoder—its imaginative powers bounded as they are to the span of just a handful of ‘respects of variation,’ the dimensions of the features vector—can imagine or conceive of whole, without approximation or simplification. Furthermore, if the autoencoder’s training was successful, the objects in the canon collectively exemplify an idealization or simplification of the objects of some worldly domain. Finally, and most strikingly, a trained autoencoder and its canon are effectively mathematically equivalent: not only are they roughly logically equivalent, it is also fast and easy to compute one from the other. In fact, merely autoencoding a small sample from the canon of a trained autoencoder S is enough to accurately replicate or model S.
From here, we climb the summit to Peli’s core claim:
• […] It is a fundamental property of any trained autoencoder’s canon that all the objects in the canon align with a limited generative vocabulary. The objects that make up the trained autoencoder’s actual worldly domain, by implication, roughly align or approximately align with that same limited generative vocabulary. These structural relations of alignment, I propose, are closely tied to certain concepts of aesthetic unity that commonly imply a unity of generative logic, as in both the intuitive and literary theoretic concepts of a ‘style’ or ‘vibe.’ […] One reason the mathematical-cognitive trope of autoencoding matters, I would argue, is that it describes the bare, first act of treating a collection of objects or phenomena as a set of states of a system rather than a bare collection of objects or phenomena—the minimal, ambient systematization that raises stuff to the level of things, raises things to the level of world, raises one-thing-after-another to the level of experience. […] What an autoencoding gives is something like the system’s basic system-hood, its primordial having-a-way-about-it. How it vibes.
“I admire the ease with which Peli moves through genres and periods, weaving between literary theory and computational scholarship, helping us, in turn, move from the knottiest aspects of machine learning to the ways literary works might learn from computation.”
Excerpt from Kathy Acker, Blood and Guts in High School, New York, Grove Press, 1984, published alongside Peli Grietzer’s “A Theory of Vibe” in Glass Bead
“Peli creates a language through which we can move back and forth from cherished humanist concepts to the impulses of experimental literature, as computational modeling helps us map language out to its edges.”
It’s a heady journey, taking many re-reads to sink in. While I wouldn’t dare to try to capture Peli’s dissertation, “Ambient Meaning: Mood, Vibe, System,” I link it here instead for all to dive into, along with an illuminating interview with Brian Ng in which the two scholars debate core concepts in the work. Peli walks through the concept of autoencoders, and the ways optimizers train algorithms for projection and compression, and does so in an inviting, clear manner. This way, when we get to the real challenges of modeling and model training, we’re prepared.
Peli notes to Ng that they understand vibe as “a logically interdependent triplet comprising a worldview, a method of mimesis, and canon of privileged objects, corresponding to the encoder function, projection function, and input-space submanifold of a trained autoencoder.” They labor to create understanding of “a viewpoint where the ‘radically aesthetic’ — art as pure immanent form and artifice and so on — is also very, very epistemic,” noting, further, the ways folks like Aimé Césaire created “home-brew epistemologies […] where the radically aesthetic grounds a crucial form of worldly knowledge.” We eventually get to an exciting set of claims about the cognitive mapping involved in attending to, as Peli writes, the “loose ‘vibe’ of a real-life, worldly domain via its idealization as the ‘style’ or ‘vibe’ of an ambient literary work,” and that, further:
• Learning to sense a system, and learning to sense in relation to a system—learning to see a style, and learning to see in relation to a style—are, autoencoders or no autoencoders, more or less one and the same thing. If the above is right, and an ‘aesthetic unity’ of the kind associated with a ‘style’ or ‘vibe’ is immediately a sensible representation of a logic of difference or change, we can deduce the following rule of cognition: functional access to the data-analysis capacities of a trained autoencoder’s feature function follows, in the very long run, even from appropriate ‘style perception’ or ‘vibe perception’ alone.
I admire the ease with which Peli moves through genres and periods, weaving between literary theory and computational scholarship, helping us, in turn, move from the knottiest aspects of machine learning to the ways literary works might learn from analogies with computation. His scholarship is as generous as it is challenging. Throughout, we’re asked to consider common metaphors and analogies used in machine learning studies. The more traditionally literary-minded are challenged to consider proof of concept in a mathematical analogy, and what artificial neural networks—not particularly the most interesting models of thought—might promise literary theory and critical studies. As we move into realms where folks are frequently moving between cognitive theoretic models, discussions of artificial neural networks, and theory, and criticism, this is a powerful guide. We’re also asked to seriously consider how literary works move towards a ‘good autoencoding’ and in what traditions of aesthetic practice we might understand aesthetics as a kind of autoencoding. Peli creates a language through which we can move back and forth from cherished humanist concepts to the impulses of experimental literature, and to appreciate computational modeling as it helps us to map language out to its edges.
“Our main task, in working together, has been discussion of each prompt around prediction and fantasies of explainability and opacity, and the roles of language in mystification and mythologizing of technology as remote.”
“As a result, the Annual is more challenging, more provocative, pushing our respondents to think along broader timescale and social scales, and challenge themselves.”
Back in April of 2020, I joined Peli for the third Feature Extraction assembly on Machine Learning, supported by UCLA, described as an exploration of the “politics and aesthetics of algorithms.” We had a fun couple of hours talking about the lifeworlds and strange logics of ML and predictive algorithms, with a group of artists and organizers at Navel in Los Angeles. I was struck, then, re-reading A Theory of Vibe three years later, how vital and alive its arguments, claims, its simultaneous experimentation and coherence, felt. In my many readings of this essay, I admired how Peli probed for unstated and unconsidered perspectives, pointed out blind spots, and how their questions were rooted in a set of exploratory hypotheses about the nature of art, and what computation allows us to see about the nature of art. They seemed a perfect interlocutor for this Annual.
As the Annual’s Research Partner, Peli has been remarkable and generous and good-humored. He is adept at the cold read, helps me make incisive cuts, always offers zoomed-out criticality. He’s helped us raise the stakes of the editorial frame, exposing us to writers and thinkers we’d not have met easily or fluidly otherwise, from computational linguists to researchers studying the computational mind to philosophers who speak through Socratic argument over our Zooms. It’s been thrilling to invite and get to know thinkers from his intense and rare circles.
Our main task, in working together, has been discussion of each prompt around prediction and fantasies of explainability and opacity, and the roles of language in mystification and mythologizing of technology as remote. As a result, the frame is more challenging, more provocative, pushing our respondents to think along broader timescale and social scales, and challenge themselves.
Over the next weeks in this Dossier, we’ll have a number of representations of our research conversations—a close-reading of Kate Crawford’s Atlas of AI, along with snippets from our conversations about the Annual—together. They’ll be gestural excerpts of the deeper conversations underway. We’re sharing ideas and discussing them in tender forms with Peli. His openness, care for thought, and a genuine enthusiasm about unexplored concepts or lesser-theorized angles has only strengthened the possibilities of this issue. Thank you, Peli!
On a final note, a massive editorial project like this Annual requires intensive research and consideration of the wider intellectual and artistic field in which the artists, writers, and thinkers invited are working. I encourage editors to try and find someone who challenges their ideas and positions, and even questions the desire to go with the safer frame. Be in conversation with someone who pushes you intellectually, who will engage enthusiastically with the deeper philosophical and critical possibilities of writing and publishing. The Annual is better for this exchange and relationship—or, forgive me—this very vibe that Peli brings.