HOLO 3: Explainability
Prompt 4: Explainability
“AI ethicists and activists frequently argue that once the black boxes of corporate algorithms are opened, users of technology will have more agency.”
“AI ethicists and activists frequently argue that once the black boxes of corporate algorithms are opened, users of technology will have more agency.”
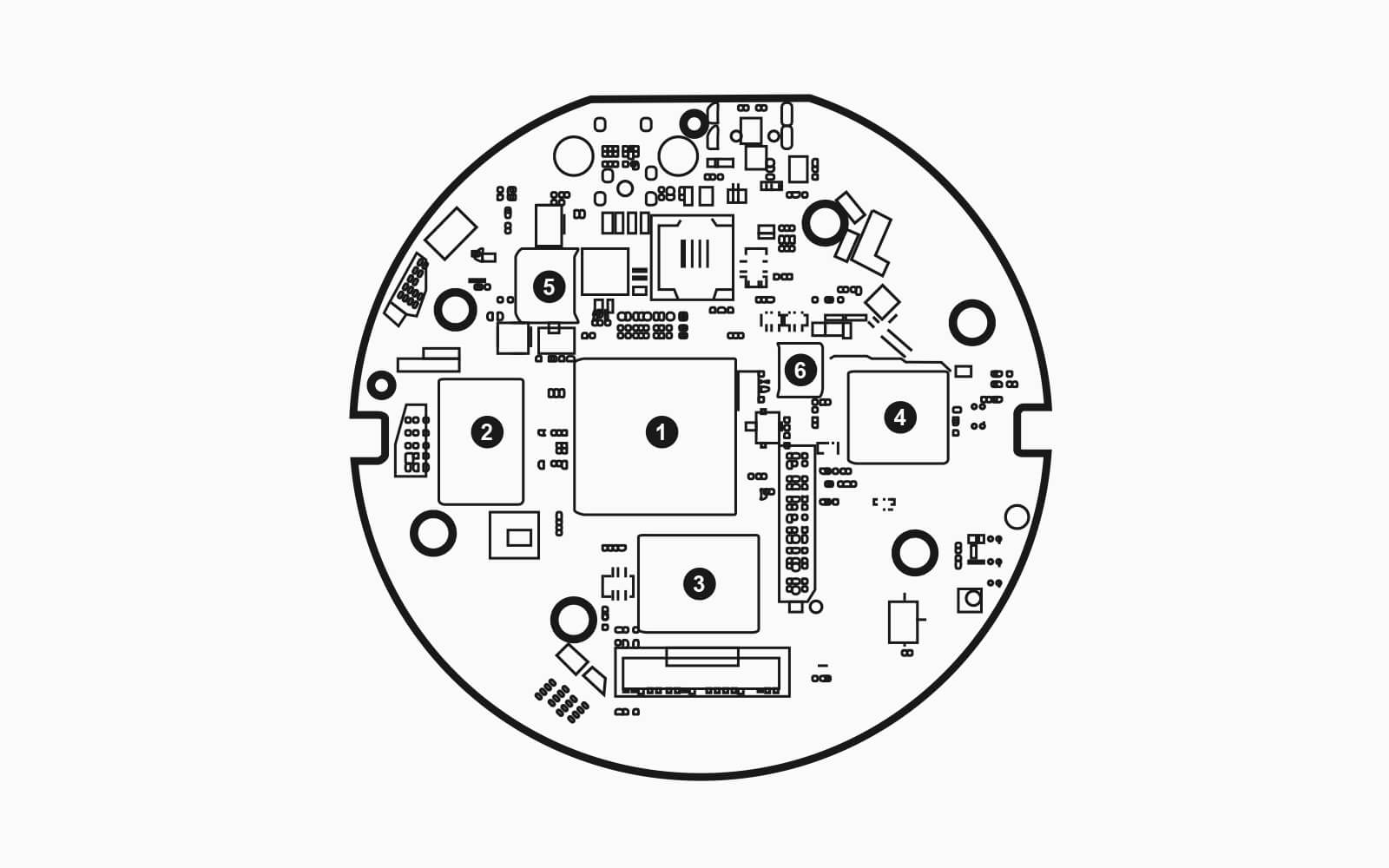

“But what happens when we don’t understand the explanation? How do we better account for ambiguity, the blurry narratives, and necessary trial and error involved?”
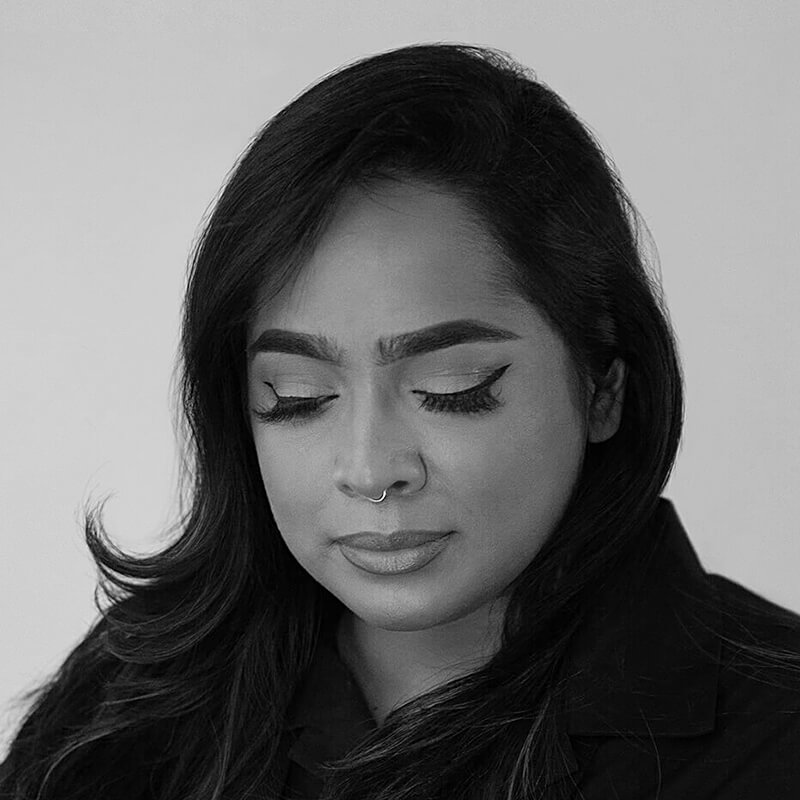
Nora N. Khan is a New York-based writer and critic with bylines in Artforum, Flash Art, and Mousse. She steers the 2021 HOLO Annual as Editorial Lead
At HOLO, the most vigorous editorial debate began with, and continued, the question of explainability. We see explainability arguments all the time around tech-; if only we meager, small-brained humans could understand all these algorithms, read them, know their every decision, we would know how to have agency in relation to them.
Explainability has massive sway as a suggested, default solution to AI quandaries, especially in relation to AI ethics. Having a more ethical dataset is contingent on knowing the data that was selected to begin with. Having an AI be able to explain its decisions, ostensibly, would mean that we have a fail-safe. The lack of explainability, or the black boxes of computation, is itself taken up as an ethical problem, a moral problem.
In a provocative 2019 debate between Yuval Harari and Dr. Fei-Fei Li, the historian and the AI researcher engage in a debate within a debate about explainability (starting around 43 minutes). Dr. Li notes that her Human-Centered AI team can give a fairly precise mathematical explanation for all the reasons for why a person might not, say, get a loan, on the decision of an algorithmic assessment. Harari notes that the answer, a statistical analysis ‘based on 2,517 data points’ weighted and measured differently might very well explain why he did not get a loan, but humans better understand decisions through flawed stories and narratives, which make the decision meaningful.
Handing the loan-denied person a set of 200 to 2,000 probability points, Dr. Li acknowledges, would be a failure on the part of the AI scientist. They then debate how a meaningful mathematical explanation, while accurate, cannot be useful for people without advanced mathematical knowledge. While Dr. Li advocates for the humanities and the arts to communicate the decisions of algorithms to the public, she also argues that explainable AI and explicability still need to be a core tenets for developing human-centered artificial intelligence.
A great number of AI ethicists and activists frequently argue that once the black boxes of corporate algorithms are opened, users of technology will have more agency in relation to AI, that human agency will no longer be undermined by intelligent tools deciding our financial and health care and social futures. Others argue that this will only improve transparency in algorithmic design.
Explainability certainly helps with understanding training methods and methods used to produce a model. Explainability can also be very helpful when we speak in counterfactuals, as when we discuss predictive or decision models. For instance, when we are rejected hypothetically as above, for a loan by an algorithmic decision, explainability demands that we get to understand why we were rejected, or how the decision would be different if the model were different. Having an explanation assumes that we get an explanation that is itself meaningful.
“How can we understand the place of artists and writers and humanists who are tasked with the ‘communication and storytelling’ that translates AI?”
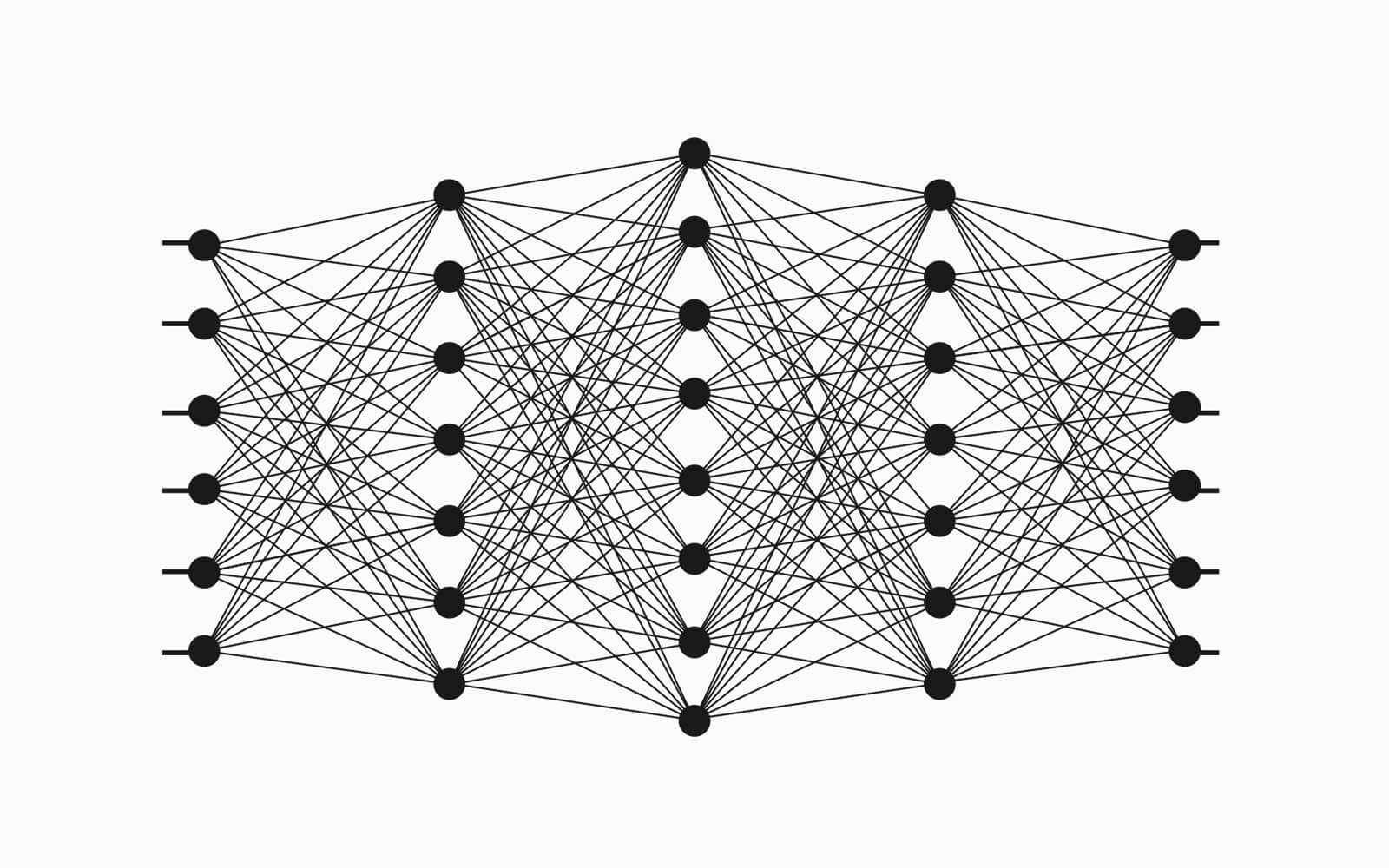
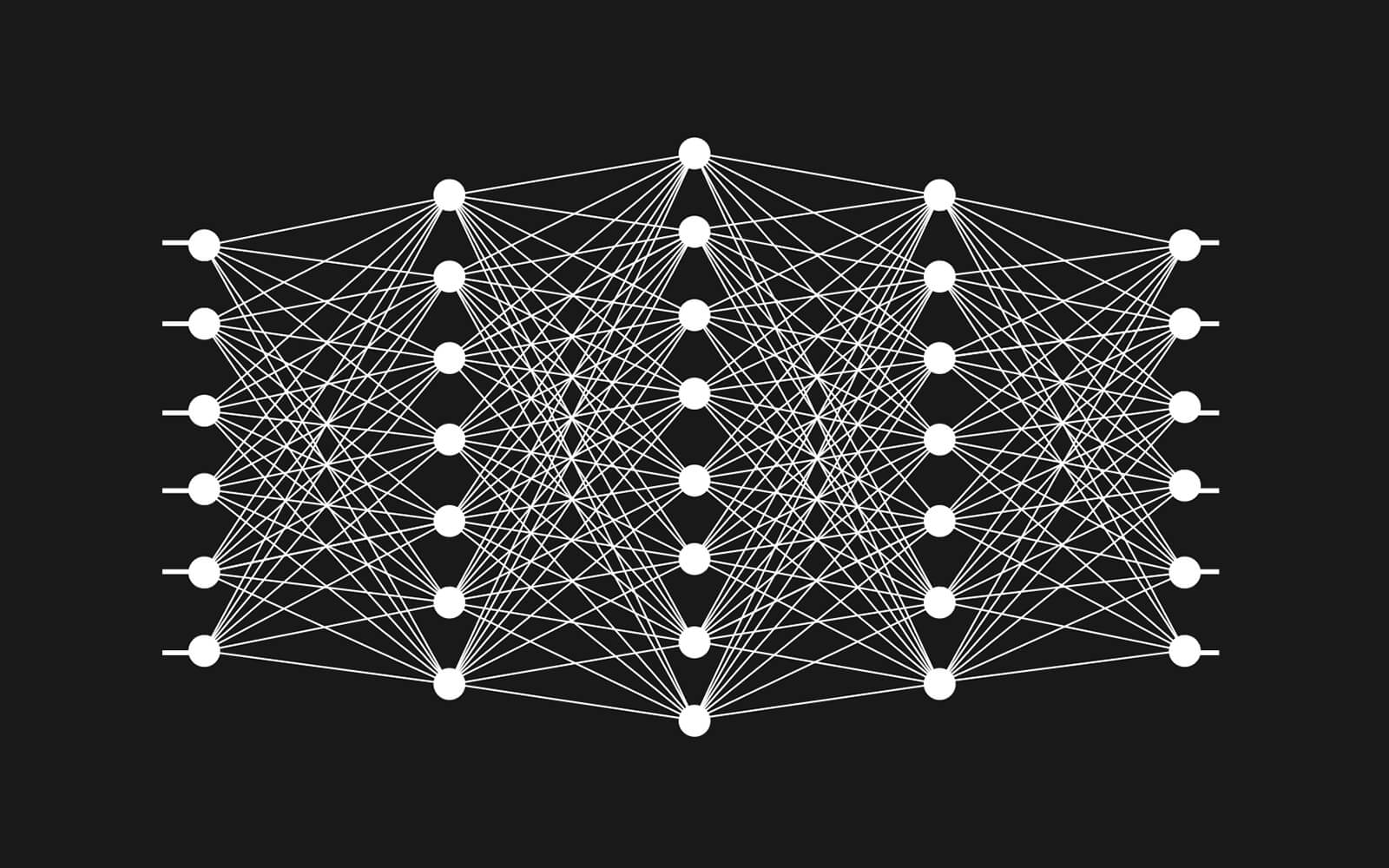
“What would explainability mean for the general public, if AI remains in service of capitalism? Can other forms of understanding inspire alternatives to the current order?”
But what happens when we don’t understand the explanation? And how do we better account for ambiguity in the scientific conversation of explainability, the blurry narratives and necessary trial and error involved? For many others, explainability is simply not enough; we need meaningful explanations, and a consideration of what is meaningful to AI, or in the space of computation, versus what is meaningful to humans as a whole, across contexts.
In this prompt, we invited thinkers to consider the many ways of explaining why a decision takes place. We shared the following questions with them:
• How do you assess situations in which algorithms take the active position, the role, of reading for the reasons for your actions, claim to know you and what you should do, better than you know? What would it mean to have a perfect ‘explanation’ of their decisions? Should algorithms be able to “explain” why they do what they do?
• How do we understand technological explainability within a tradition of discursive rationality, or the desire for deep learning to explain itself in the way that a person explains itself? How might we think of this desire for justification, a kind of accountability, as a fantasy rooted in an analytic philosophical exchange? (How do we square this desire for exchange with an AI working at scale, involved in massively probabilistic thinking)?
• Further, what is lost in the desire for total explainability? What other models of explainability and understanding do we need to have at play in discussing AI or black-boxed technologies? What are some competing models for explainability, outside of scientific understanding of a process, and counterfactuals about a predictive process?
• How can we understand the place of artists and writers and humanists who, as Dr. Li notes, are tasked with the “communication and storytelling” that translates AI? What are other ways of understanding that form alternatives to the loops of critique of inexplicability? What would legibility and explainability mean for the general public, if AI remains in service of capitalism, and the accrual of state capital?
• What are other forms of ‘explanation’ or understanding of AI that would help us think about alternatives to the current order, rather than investing in more critique of AI within the current order?
Intrepid authors, thinkers, and artists tackle the philosophical and aesthetic dimensions of this prompt: Jenna Sutela, Sera Schwarz, Ryan Kuo, and Ingrid Burrington. They’ve created maps of explanation and created deep dives into what it would mean to have a meaningful explanation. Get to know them below, and then enter their arguments and mappings in HOLO 3:
Ingrid Burrington writes, makes maps, and tells jokes about places, politics, and the weird feelings people have about both. Much of her work focuses on mapping, documenting, and studying the often-overlooked or occluded landscapes of the internet (and the ways in which the entire planet has become, in effect, a “landscape of the internet”). She is the author of Networks of New York: An Illustrated Field Guide to Urban Internet Infrastructure. Burrington’s work has previously been supported by Eyebeam, Data & Society Research Institute, the Studio for Creative Inquiry, and the Center for Land Use Interpretation.
Ryan Kuo lives and works in New York City. His works are process-based and diagrammatic and often invoke a person or people arguing. This is not to state an argument about a thing, but to be caught in a state of argument. He utilizes video games, productivity software, web design, motion graphics,and sampling to produce circuitous and unresolved movements that track the passage of objects through white escape routes. His recent projects aim to crystallize his position as a hateful little thing whose body fills up white space out of both resentment and necessity. These include any conversational agent that embodies the blind “faith” that underpins both white supremacy and miserable white liberalism and casts doubt on non believers, and an artist’s book about aspirational workflows, File: A User’s Manual, modeled after software guides for power users.
Sera Schwarz is a writer and philosopher based in Berlin. Their work moves between the philosophy of psychology, epistemology, and ethics, and focuses on the points of contact (and conflict) between perspectives on the mental underwritten by the contemporary cognitive sciences and the humanist tradition. They are currently a graduate student at the Berlin School of Mind and Brain, Humboldt-Universität. They also write poetry and make sound art.
Jenna Sutela works with words, sounds, and other living media, such as Bacillus subtilis nattō bacteria and the “many-headed” slime mold Physarumpolycephalum. Her audiovisual pieces, sculptures, and performances seek to identify and react to precarious social and material moments, often in relation to technology. Sutela’s work has been presented at museums and art contexts internationally, including Guggenheim Bilbao, Moderna Museet, Serpentine Galleries, and, most recently, Shanghai Biennale and Liverpool Biennial. She was a Visiting Artist at The MIT Center for Art, Science & Technology (CAST) in 2019-21.